What is the greatest legal movie ever, according to lawyers?
Any guesses?
12 Angry Men? To Kill a Mockingbird? A Few Good Men?
Nope!
The correct answer is My Cousin Vinny, a movie about a new lawyer named Vincent Gambini who travels from New York to Alabama to defend his cousin in a murder trial. The movie revolves around Gambini and his fiancee trying to get through the trial (his first ever) while dealing with a hostile judge and an utter inability to get a good night’s sleep.
Seriously, lawyers love it. A federal district court judge once wrote that the creators of the movie had “given our profession a wonderful teaching tool while producing a gem of a movie that gives the public at large renewed faith in the common law trial and the adversarial system as the best way to determine the truth and achieve justice.”
Well, if it’s good enough for them, then it’s good enough for me!
I, like Vincent Gambini, am operating on very little sleep right now (attending Money20/20 and then returning home to toddlers isn’t conducive to rest), so I figured I’d use quotes from My Cousin Vinny to assist me in breaking down a fintech topic that I’ve been meaning to write about for a while – alternative data.
Ready? Let’s go!
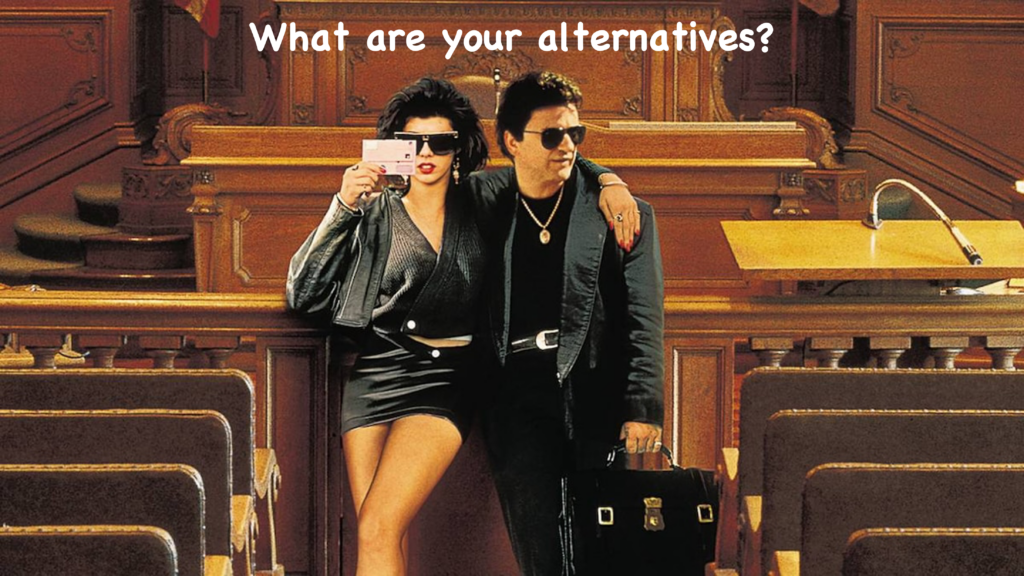
“Everything that guy just said is bullshit. Thank you.”
The best opening statement in legal movie history also explains why I am writing (ranting?) about alternative data.
Industry commentators love to talk about alternative data. It is often presented as a panacea to all the ills in consumer lending, a thing that (when paired with machine learning and AI) can produce alchemical-level results.
The vast majority of this commentary is, if we’re being honest, bullshit.
“I understand, but ya know, what are your alternatives?”
A big problem with a lot of the commentary around alternative data is that it’s maddeningly unspecific.
Like, what exactly is alternative data?
I found this recent answer from Tom Hadley on Twitter amusing:
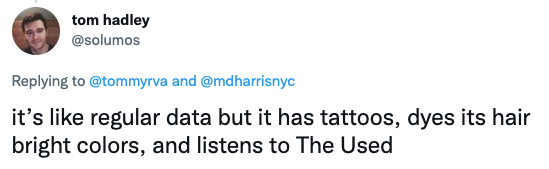
I think this answer is also instructive because it suggests that being ‘alternative’ requires that you be in contrast to something (presumably something mainstream … the clean-cut data that never talks back to its parents and thinks pop music is perfectly cool).
Join Fintech Takes, Your One-Stop-Shop for Navigating the Fintech Universe.
Over 36,000 professionals get free emails every Monday & Thursday with highly-informed, easy-to-read analysis & insights.
No spam. Unsubscribe any time.
With that framing in mind, I would define alternative data like this:
Alternative data is data that has not traditionally been captured by the big three credit bureaus, and that is used to evaluate a consumer for a lending product.
In other words, alternative data is credit data that sits outside the credit bureaus.
Getting even more specific, I think it’s important to draw distinctions between different types of alternative data in terms of both data type and proximity to the traditional credit file:
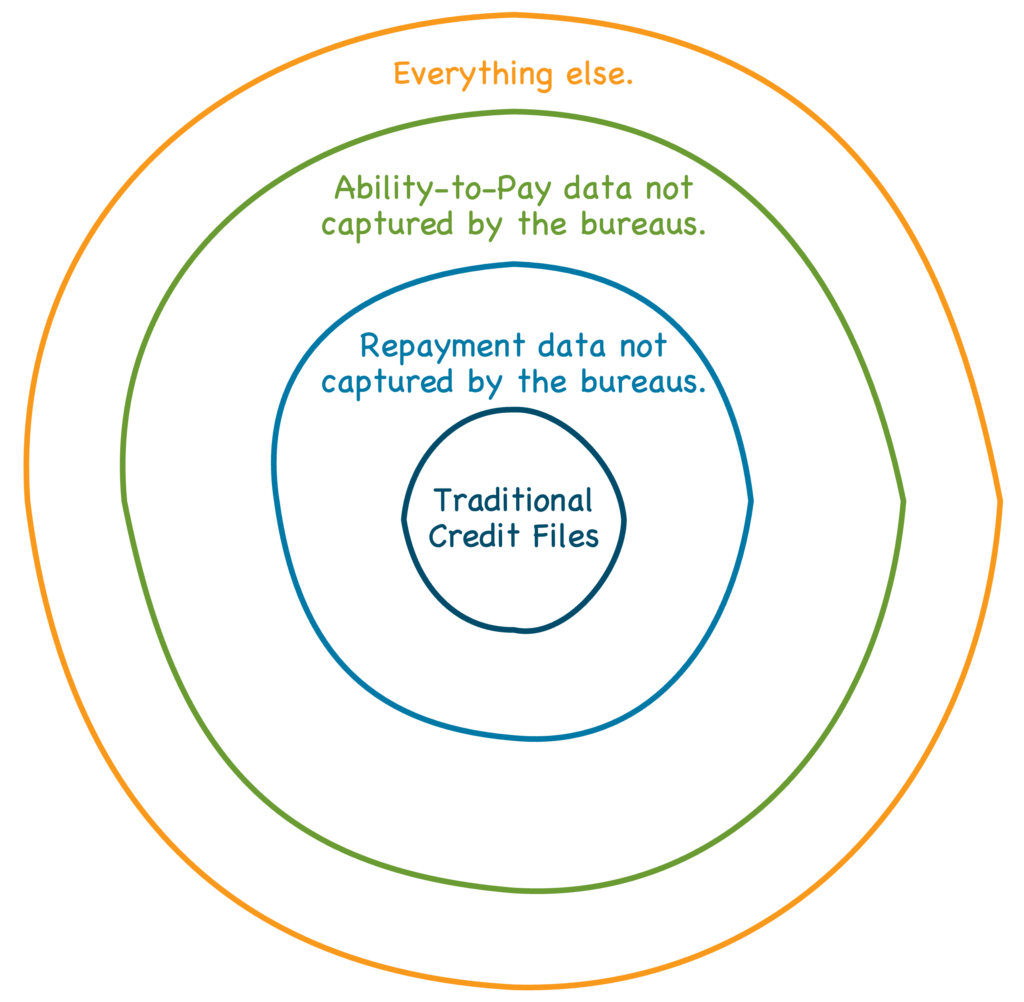
The center of the target – traditional credit files – is comprised of repayment data for traditional lending products and relevant public record data (liens, bankruptcies, etc.)
The next ring in the target is repayment data that has traditionally not been included in the big three’s credit files. That could be repayment data on non-loan obligations (rent, utilities, etc.) or repayment data for new or more exotic loan product types that the mainstream credit bureaus are less accustomed to dealing with (BNPL, subprime loans, etc.) Much of this alternative repayment data has been getting gobbled up by the big three credit bureaus over the last 10-15 years, and that trend has accelerated as fintech companies focused on credit building have appeared and assisted consumers in furnishing more of this data to the bureaus.
The next ring in the target is ability-to-pay data. This is a fundamentally different type of data signal for lenders, which can be complementary to the ‘willingness to pay’ signal that traditional and alternative repayment data provides. An increasingly common type of data in this category is cash flow data from consumers’ deposit accounts. Experian, Equifax, and TransUnion have shown an interest in helping lenders use this data in addition to their traditional ‘willingness to pay’ data, but it’s usually treated and organized as a distinct type of data asset that is often, though not always, captured by other companies besides the bureaus.
Finally, the outer ring is everything else – literally any other piece of data that could, in theory, be used to help a lender predict if a potential customer would be likely to repay them. This is always-sounds-compelling-in-theory-but-is-rarely-that-useful Big Data category. It includes everything from data on how many times a consumer calls their parents to how frequently they misspell words in their social media posts to their age, gender, and race.
“Are you sure?”
“I’m positive.”
“How could you be so sure?”
How a consumer has repaid loans (and, to a lesser extent, other recurring bills) in the past is, by a country mile, the most useful data point for predicting if they will repay a loan in the future.
Am I sure about this?
Yes, I’m positive.
How can I be so sure?
Well, lenders have tested EVERYTHING extensively, and nothing else has performed nearly as well as past repayment behavior.
Even if you take the regulatory leash off and really let data scientists go crazy and use any and all Big Data assets that they want to try and construct a better-performing model, they still won’t find a better way to predict loan repayment.
Again, how do I know this?
They tried it in China (emphasis mine):
When Ant Financial launched its credit scoring system, Sesame Credit, in January 2015, it said the data-driven product would “make credit more available to millions of consumers across China”, giving individuals access to everything from mortgages to mobile phone contracts to car loans.
But nearly four years later, Ant Financial, which is an affiliate of Chinese tech giant Alibaba, has never used Sesame Credit for lending decisions, and critics are increasingly questioning whether the tool can be used to accurately assess individual behaviour.
Sesame, which is an opt-in feature of the Alipay mobile payments app, draws upon the biggest pool of non-traditional ratings data in the world. It synthesises details from hundreds of sources — ranging from purchases on Alibaba’s Taobao marketplace to subway fares — into a single trustworthiness number for each user, called a “Sesame score”.
But one Ant Financial employee conceded there was a difference between “big data” and “strong data”, with big data not always providing the most relevant information for predicting behaviour, and analysts say the best predictor of whether someone will default on a loan in future is often their previous loan repayment history, rather than their likelihood of returning a rental car.
“Would you like me to explain?”
The vast majority of Big Data is of little-to-no use in predicting borrower default, but to the extent that it is theoretically useful, we’ll never be able to use it in the U.S. anyway.
One of the central tenets of our consumer lending laws and regulations is the belief that if a consumer is declined for a loan, they deserve to know why. This is the reason that the credit bureaus (and any data provider subject to the Fair Credit Reporting Act) are required to provide declination reasons to consumers (this is why you weren’t approved) and why consumers have the right to see their own credit files and dispute any inaccuracies.
We want people to be able to understand what they did wrong and have the ability to take action to correct it.
This is an underrated feature of our current lending system – it’s really hard to game. If a lender tells a consumer that they were declined because of excessive late payments, and then the consumer goes, “ohh yeah? I’ll show you! I’ll pay on time for the next 36 months! You’ll see! You’ll all see!” That would be an objectively good thing. The consumer would be altering their financial behaviors in a way that wouldn’t just make their score go up, it would actually make them a less risky customer for the next lender they apply to.
When you use a Big Data variable that is loosely correlated with default risk but isn’t logically related to a consumer’s financial behaviors, you put yourself in a tough position. You can use the variable – let’s say it’s the prevalence of ALL CAPS typing in social media updates – to help you decide not to loan a consumer money, but you absolutely can’t tell them why they were declined without giving them an easy-to-follow roadmap for gaming your algorithm (just stop typing in ALL CAPS).
“Oh yeah, you blend.”
People are getting very excited about cash flow underwriting based on bank account transaction data.
And for good reason! Modern, consumer-permissioned data aggregation can empower consumers without traditional credit files to access entry-level credit products (low-limit credit cards, small-dollar personal loans, etc.)
However, it’s important to be clear – cash flow data is not a replacement for traditional credit data, and it never will be.
Why?
Cash flow data helps lenders understand a consumer’s ‘ability to pay’, which is to say, the amount of money that the consumer makes and spends and the delta between the two.
Traditional credit files (and credit scores like the FICO Score) help lenders understand a consumer’s ‘willingness to pay’, which is to say, how the consumer prioritizes the repayment of debt.
There is an obvious relationship between these two variables – a consumer with a great track record of paying back loans on time may still default on a loan if they suddenly lose all of their money. As such, cash flow data can be used to help modulate risk of default predictions made using traditional credit files and scores
However, from a statistical perspective, “ability to pay” data and “willingness to pay” data are orthogonal to each other. One cannot replace the other. Instead, smart lenders will blend both signals into their models, letting each of them do what they do best.
(Quick sidenote – this is one of Marisa Tomei’s first lines in the movie, and My Cousin Vinny was her first big break. She actually won an Academy Award for Best Supporting Actress for her performance, which was completely deserved. She basically pitches a perfect game in this movie.)
“So, as your eyes become more and more out of whack, as you’ve gotten older, how many levels of thickness have you gone through?”
“I don’t know, over 60 years, maybe 10 times.”
“Maybe you’re ready for a thicker set.”
In honor of the best cross-examination montage in legal movie history, I want to spend a minute cross-examining an argument about alternative data that I’ve been seeing pop up more and more.
The National Consumer Law Center (NCLC) recently published an article arguing that the reporting of rental payment data to the bureaus might be, on balance, a bad thing for consumers:
Rent payment data has been aggressively promoted as a form of alternative data that will help consumers who are either credit invisible or have poor credit histories. In the wake of the racial justice movement of the past few years, rent reporting has been embraced by industry, policymakers, and some nonprofits as one of the primary ways to help Black and Latinx consumers, given the stark racial disparities in credit scores and credit invisibility.
However, rent reporting actually carries huge risks for renters, especially the most vulnerable families who struggle with housing costs. These at-risk households are also disproportionately Black and Latinx. Rent reporting risks helping some better-off credit invisible consumers at the cost of literally making other renters homeless.
The article goes on to argue that rather than reporting positive and negative rental payment history to the credit bureaus (so-called ‘full file reporting’), only positive data should be furnished to the bureaus:
The most important consideration is that rent reporting should be limited to positive payment information only. Programs that report negative or “full file” information will most certainly harm vulnerable, struggling families. This is because landlords use credit reports and scores, either independently or bundled with criminal history and eviction records as part of tenant screening reports. Many landlords will not rent to a consumer with any record of a late rent payment, or will charge them a prohibitively high security deposit.
I don’t agree.
I get where the authors of the article are coming from (and I agree with the goal they are optimizing for), but the answer is not to keep out negative data.
Any data that is furnished to lenders for the purposes of making a credit decision needs to be comprehensive. It needs to include both the good and the bad. If we allow consumers to cherry-pick the data that they allow lenders to see about them, we will reduce the ability for lenders to price risk accurately, and the end result will be less access to affordable credit for everyone.
There’s a better solution …
“Mr. Gambini, the next words out of your mouth better be “guilty” or “not guilty.” I don’t want to hear commentary, argument, or opinion. I don’t want to hear any facts or evidence. If I hear anything other than “guilty” or “not guilty”, you’ll be in contempt. I don’t even want to hear you clear your throat to speak. Now, how do your clients plead?”
I’ll keep this simple, as Judge Haller instructs us to – it should not be legal to use credit bureau data or credit scores to make any decision except whether or not to lend someone money.
Insurance carriers shouldn’t be able to see my credit report. Nor should prospective employers or landlords.
I actually have no idea why we allow this. And if we stopped allowing it, it would solve the problem that the NCLC rightly flagged above.
“All I ask is for that one chance. I think you should give it to me.”
It’s important in any discussion about the relative merits of different alternative data types to remember that consumers (the ones we are trying to serve) are generally very in favor of lenders using any and all types of data in order to approve them for a loan. This finding from a survey conducted by Pinwheel supports this point:
More than 75% of people working in America believe a credit score should not be the only criterion for getting a loan. Half of the respondents stated the credit scoring system is missing information that shows they are financially responsible: income, utility bill payments, phone bill payments, and bank account balances were the top suggested alternative criteria.
(BTW – if anyone at Pinwheel is reading this – I’d love to get a sneak peek at the full results from this survey )
“No, I hate him”
In his recent comments on open banking and Dodd-Frank Section 1033, the Director of the CFPB made it clear that the bureau was going to do what it could to hobble centralized, rent-seeking middlemen:
we are exploring safeguards to prevent excessive control or monopolization by one, or even a handful of, firms. A decentralized, open ecosystem will yield the most benefits for creators and consumers alike. At the same time, there will be strong incentives for gatekeepers and intermediaries to emerge, extract rents, and self-preference. In consumer financial services, we have a number of highly concentrated submarkets: the credit reporting conglomerates, the card networks, the core processors, and more. It’s critical that no one “owns” critical infrastructure.
Translation? We don’t like the big three credit bureaus, and we won’t like any new centralized data aggregators that end up playing a similar role in the industry.
As the credit bureaus continue to try to gobble up more sources of alternative data and open banking aggregators like Plaid and MX sharpen their focus on lending and lending-adjacent use cases (just an educated guess on my part!), it will be interesting to see what the CFPB does.
“Excuse me, you guys down here hear about the ongoing cholesterol problem in the country?”
The discussion around alternative data often intersects with another, more important conversation – the ongoing discrimination that women and people of color face in getting access to affordable credit.
There are two important points to touch on here:
1.) Alternative repayment data (rent, utilities, etc.) and ability-to-pay data are correctly seen as useful tools for increasing access to affordable credit for women and people of color who might not qualify based solely on their traditional credit histories.
2.) The use of data from the ‘everything else’ category also has the potential to help lenders make fairer decisions, although this is an area of robust debate and disagreement. A good example is the use of identity-based data variables like race and gender. Since the passage of the Equal Credit Opportunity Act in 1974, the use of these variables in lending has been strictly prohibited. However, some argue that the use of these variables in training underwriting models might actually reduce discriminatory outcomes. Here’s Kareem Saleh, Founder and CEO of FairPlay:
Many underwriting models look for consistent employment as a sign of creditworthiness: the longer you’ve been working without a gap, the thinking goes, the more creditworthy you are. But if Lisa takes time out of the workforce to start a family, lending models that weigh “consistent employment” as a strong criterion will rank her as less creditworthy (all other things being equal) than a man who worked through that period.
The result is that Lisa will have a higher chance of being rejected, or approved on worse terms, even if she’s demonstrated in other ways that she’s just as creditworthy as a similar male applicant.
Models that make use of protected data during training can prevent this outcome in ways that “race and gender blind” models cannot. If we train AI models to understand that they will encounter a population of applicants called women, and that women are likely to take time off from the workforce, the model will know in production that someone who takes time off shouldn’t necessarily be deemed riskier.
(This scene in the movie, where Vinny and Mona Lisa are having breakfast at the diner, isn’t really critical to the plot, but it is great. The way that the menu that only lists one option for breakfast – ‘Breakfast’ – flummoxes them is just perfect.)
“They didn’t teach you that in law school either?”
As the tools that lenders use to make credit decisions become more sophisticated – the AI hype train is coming through! Choo choo! – and we start incorporating new and even previously-restricted data variables like race and gender, we are going to need a regulatory infrastructure that can keep up.
Having witnessed the process that bank regulators use today to evaluate lending compliance up close a few times, I have to say that I’m not super optimistic.
“Hey, Stan, you’re in Ala-fuckin’-bama. You come from New York. You killed a good ol’ boy. There is no way this is not going to trial!”
Let’s end with the best quote in the movie.
When it comes to innovation in consumer lending, alternative data (and AI/ML) gets all the headlines.
However, I think the much more impactful shift in how lending is done will come about as control of the data that lenders use moves from lenders (and centralized data aggregators like the bureaus) to consumers.
The momentum around cash flow underwriting and the use of bank account data is real. From an adoption perspective, it is actually outpacing the use of alternative repayment data like rental and utility data. That is according to a recent survey of lenders from Nova Credit:
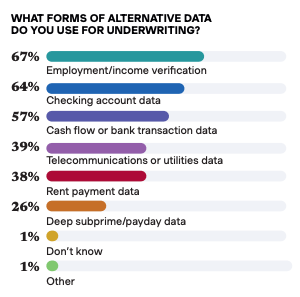
This momentum is important because it helps move consumer-permissioned data access closer to becoming the standard in the industry and, as a consequence, significantly shifts the power for how lending decisions get made.
It’s the consumers’ data. The CFPB agrees. The credit bureaus have been monetizing it without consumers’ consent. There is no way this is not going to trial!