I sometimes feel like I’m the fintech equivalent of a therapist.
Founders, operators, and investors will tell me what they’re working on, what’s going well, and what’s not going well. And then, individually or through the newsletter, I will carefully try to reflect what I’m hearing back to them, subtly trying to reframe certain concepts or challenge their thinking in different ways. I try not to give specific advice because I don’t want to substitute my judgment or values for theirs.
99% of the time, I think this is the right approach.
However, in the same way that I imagine therapists occasionally want to skip all the back and forth and just tell their patients the right answers, there are times when I want to be direct. I often feel this way when the subject of the discussion is lending, as that is an area that I know extremely well, and see the same mistakes being made over and over.
So … you know what?
In today’s essay, I’m going to do precisely that. I’m just going to give you the answers.
(Editor’s Note — Yes, I have seen the TV Show “Shrinking,” and this essay is very much modeled after Jimmy’s unconventional approach. Let’s call it Alexing.)
The subject for today’s essay is small business lending, a topic I have written about multiple times (most recently here and here). And to help me with this exercise, I have recruited Alex McLeod. Alex is the Founder & CEO of Parlay Finance, a fintech infrastructure company focused on small business lending.
Alex (who I will refer to as “McLeod” from here on out to minimize confusion) and I are going to take turns sharing fundamental observations about lending to small and medium-sized businesses (SMBs), which are (you’ll just have to trust us) true.
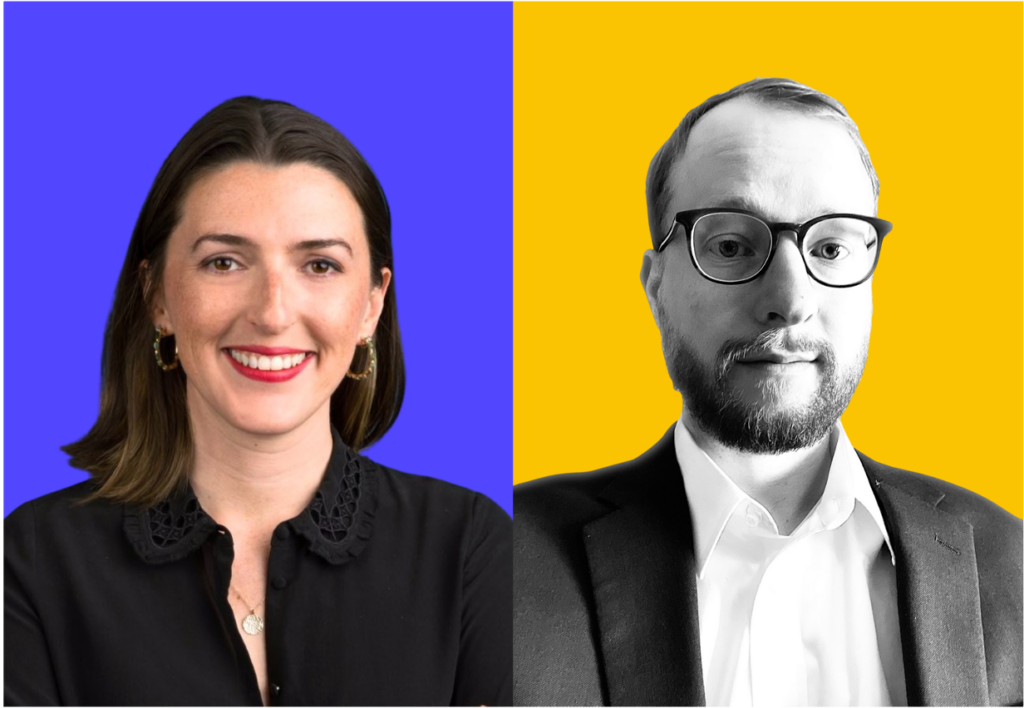
Johnson: McLeod, you go first.
McLeod: I’d love to start with a dose of reality for America’s community lenders. Megabanks and fintech lenders are coming after your relationships with small business owners!
Incremental modernization — updating one system at a time — is no match for the giant technological leap we’re making with AI-powered systems. You can’t win a race by walking faster when your competitors are driving cars.
Historically, community lenders won by being relationship-driven, understanding their local markets in ways bigger institutions never could. But the market has shifted dramatically. Business owners now want instant, digital everything — clean online applications, quick decisions, and minimal friction. Legacy lending practices are too expensive and inefficient to meet these demands. The only way forward is to leapfrog into leveraging automation and AI in a way that lets lenders build relationships, manage risk, and scale operations effectively.
As someone building in this space, I’m genuinely excited about AI-powered SMB lending, but I am worried that most lenders do not understand these capabilities well enough to harness their incredible potential while maintaining safety and soundness.
It’s like the parable of the blind men and the elephant. A lot of people think they have ‘AI for lending’ figured out, but almost no one sees the whole picture. For example, you have some lenders who think basic automation is enough — they’re celebrating three-yard gains when they automate document collection and basic underwriting, thinking they’ve scored the touchdown. On the other end, there’s this gold rush mentality where lenders think AI is the silver bullet that will magically solve everything in lending. Both miss the point. Smart lenders understand that AI isn’t just about digitizing processes but about using transformative technology to better serve customers.
In this new era of AI-powered SMB lending, we need to return to first principles that allow bankers to harness these tools while mitigating new risks.
What’s your take, Johnson?
Johnson: I think you are right about the accelerating pace of competition in small business lending and the threat that it poses to community banks and credit unions. And you are speaking my language when you talk about a return to first principles. I wrote a whole essay on that exact subject — A First Principles Look at Small Business Lending.
The basic reality, which we need to be honest about, is that small business lending is really freaking hard.
And this leads me to my first small business lending truth bomb: one model can’t rule them all.
The success of FICO and the standardization of credit risk underwriting for consumer lending has led many smart people to assume that they can build the equivalent unified decisioning model for small business lending.
None of them have succeeded.
Why?
A restaurant’s January financials look nothing like July’s. A construction company’s mid-project position barely reflects its completion state. Comparing two businesses from completely different industries, sizes, business models, or geographies is like comparing apples to kangaroos. Unlike consumer lending, where risk can be approximated for millions of similar applicant profiles using metrics like credit scores, small business lending is blessed and cursed with infinite variety.
This is why I believe the future of SMB lending will require hundreds of specialized models calibrated against every possible combination of industry, loan type, and business model. These models won’t be built through old distribution networks (community banks dominating SMB lending through geographically concentrated branch networks) but rather by vertically-focused software platforms that focus on creating “operating systems” for SMBs in specific industries.
A good example is Toast. The company has become a monster in the SMB restaurant lending space. Couldn’t you envision Toast building a credit score for restaurants, which other lenders (like community banks and credit unions) could leverage?
Have I wandered too far outside the box, McLeod?
McLeod: You’re right on target — banks serving SMBs must prepare for a future dominated by hyper-segmented, personalized lending products that bypass the rigid credit boxes of yesteryear. But…
Before we can get to that future filled with hundreds of specialized models, we need to grapple with a terrible truth: SMB lending has a horrific data problem.
Most lenders today fail to capture their applicant data in clean, structured, real-time formats, let alone build specialized models from it.
It gets worse — community lenders are often held hostage by Frankenstein product stacks that are better at creating data silos than surfacing opportunities to create customer value. These problems are usually compounded by workflows that trap, or lose, data from manual processes, phone calls, and emails.
This mountain of trapped and missing data means lenders are trying to compete without the insights they need to succeed.
Until lenders fix this data collection and structuring problem, they cannot embrace AI capabilities or train models that support their unique business needs. This means they fall further behind competitors and customer expectations each day. Even the smallest community lender is missing out on thousands of valuable insights that could unlock growth and help them better serve small businesses.
However, if you have the right intake systems, you can build proprietary datasets (and models!) on small business behavior, creditworthiness, and loan readiness that nobody else has. That’s a gold mine. There’s your future competitive advantage as a lender. But until we solve the data problem, the old adage stands: garbage in, garbage out.
I’ve been deep in the weeds on this next question, Johnson, but I’m curious about your perspective: once we solve the data foundation problem, where do you draw the line between what should be automated, and what needs to stay human-driven?
Johnson: I’m so glad you asked!
This is another area where commercial lending fundamentally differs from consumer lending — the ideal amount of human involvement is not zero!
In consumer lending, borrowers want you to get out of their way. A loan is a tool to get something (an iPad, a car, a house, etc.) Consumers want to go as little out of their way as possible to get that thing. They don’t want to talk to your loan officer, no matter how charming and helpful they are.
Commercial lending is different.
Businesses don’t get loans for pleasure. They get them for … well … business.
Commercial lending is consultative. Companies’ need for additional capital is almost always strategic (I need to grow) or existential (I need to save my business from dying). In both cases, commercial borrowers tend to want their loans bundled with expert advice and guidance.
Now, of course, just how much human involvement is needed or can be economically justified in the commercial lending process depends on how big the commercial entity is and how much money it needs.
A $2 million commercial real estate loan is a bespoke product assembled by relationship bankers. That’s what the borrower wants (being wined and dined by bankers is fun … I’ve been told), and it’s what the economics of the deal can support. We’ll see some automation applied around the edges (indeed, we already are), but humans will likely always play a starring role.
A $50,000 working capital loan to a small business is an entirely different matter. The need for these types of loans tends to be more existential, and, as such, underwriting and funding speed are critical. Automation (built on a solid data foundation!) is incredibly important.
However, I should note that automation in small business lending doesn’t, by itself, win the day. I know this because the last generation of SMB-focused fintech lenders (Kabbage, OnDeck, etc.), while disruptive thanks to their innovative use of automation and digital channels, weren’t as successful as many industry observers (including me) predicted they would be. Surveys of small business owners have consistently shown that while speed and convenience are important factors for deciding where to get a loan, the quality and personalization of the service that small business borrowers receive tend to be the most important factors for determining how satisfied they end up being with the loan.
True staying power in small business lending requires at least some level of expert advice and guidance.
My question for you is this: Will humans always be needed to provide that guidance, or can it come from an algorithm?
McLeod: That’s a fascinating question. My take is that different lending problems need different AI solutions.
Generative AI is a good example. We’ve decided to treat it as the silver bullet, but why? You wouldn’t use a hammer for every home repair; why solve every lending problem with the same type of AI?
We must match the right use case to the right models using the right data.
Want to automatically intake and sort leads by loan eligibility? That’s perfect for a machine learning classification model.
Want to uncover new customer micro-segments for tailored product offerings? Try unsupervised clustering algorithms.
Need to provide readiness insights or guidance? Try a recommendation engine.
Dealing with those tricky edge cases where a deal structure needs tweaking? Agentic AI could help, with human oversight.
Plus — and this is my final thought — automation is a spectrum, not a switch. As you said, we are probably never going to 100% automate small business lending. You don’t want algorithms recommending bad actions or advice. Human judgment still adds irreplaceable value.
If I’m a small business in that scenario you mentioned before – “I need to save my business from dying” – I won’t be consulting a chatbot.
Johnson [using his fintech therapist voice]: Well, that’s all the time we have for today!