FICO is one of the most successful fintech companies ever. Since the release of the first general-purpose credit score — the FICO Score — in 1989, which transformed credit scoring from a one-off consulting business (with high incremental costs) to a SaaS business (with almost zero incremental costs), the company has been on a tear. Here’s how I described it in an essay last year:
At the time FICO IPO’d in 1989, the company generated just $18 million in revenue. By 1995, it was $114 million, with a significantly higher profit margin, thanks mainly to the FICO Score and the company’s distribution arrangements with the credit bureaus and major credit card processors.
And in 1995, FICO was blessed by the government with more good fortune – the FICO Score became the standard credit scoring model used to underwrite and securitize conforming mortgages (i.e., mortgages that can be sold to Fannie Mae and Freddie Mac). By 2000, 75% of all mortgage applications in the U.S. were decisioned using the FICO Score.
The result of all these developments has been an incredible 35-year run, with FICO’s stock compounding 20% year over year since its IPO and the company maintaining a hold on roughly 80-90% of the consumer credit scoring market in the U.S.
To put it more simply, FICO is the fintech equivalent of Google. It took a genuinely innovative idea (statistics-based credit evaluation for FICO and link analysis-based search for Google) and transformed it into an unprecedentedly efficient engine of profitability (general-purpose credit scores for FICO and online advertising for Google).
The FICO Score is the exact type of business that everyone wants to build. The problem is that opportunities to build businesses like that don’t come around very often, and when they do, everyone goes after them.
That’s one of the reasons why folks in and around the lending ecosystem are so excited about cash flow data.
Disclosure – The remainder of this essay is about cash flow-based lending analytics. It’s not really about FICO, but (as you’ve already seen) it will discuss this topic in the context of FICO and the FICO Score. I worked at FICO, on the software side of the business, between 2016 and 2021, and I have worked for more than 20 years in close proximity to FICO and the credit bureaus. As is eternally the case here at Fintech Takes, nothing I write is based on proprietary information, nor should it ever be construed as investment advice. on is that the “how” matters just as much as the “what” when it comes to turning campaign promises into government policy.
The Cash Flow Opportunity
I’ve written a lot about cash flow lending in the newsletter over the last few years (most notably here, here, and here), so I won’t belabor this point too much, but suffice it to say that the emergence of open banking in the U.S. has created a once-in-a-generation opportunity to tear apart and rebuild the infrastructure that lenders rely on to make credit decisions.
The reason this is true is that consumer-permissioned bank transaction data gives lenders a timely and highly accurate view of prospective borrowers’ cash flow history and patterns. And this insight is enormously helpful, both in addition to and (in some cases) in place of traditional credit data, for predicting loan defaults.
So, the race is on to rebuild every part of the traditional credit decisioning “stack” so that it can accommodate the unique benefits — and challenges — of consumer-permissioned bank transaction data.
Before we take a look at some of the specific companies running in that race, we first need to ground ourselves in an understanding of how that stack works today (and why FICO holds such a privileged position in it). The irony is that these outcomes are exactly the opposite of President Trump’s stated goal of reviving American manufacturing, as Derek Thompson points out:
The Credit Decisioning Stack
To oversimplify a bit, the traditional credit decisioning stack is comprised of three blocks — a data repository (like a credit bureau), an analytics provider (like FICO), and a decision engine (like my first employer, Zoot Enterprises). The lender and the customer sit on top of the stack, and everything flows upwards; the data repository supplies credit data, the analytics provider uses that data to calculate attributes and scores, the decision engine takes in those attributes and scores and outputs a decision, and (if the application is approved) the lender presents an offer to the customer.
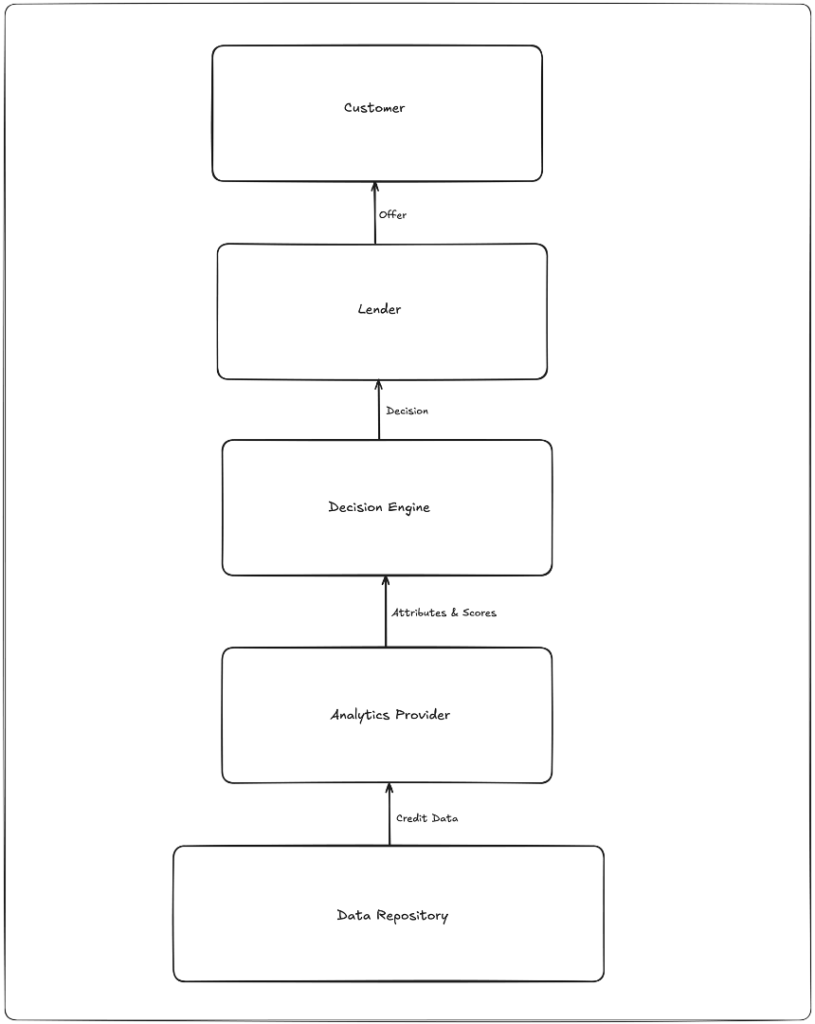
There are two additional layers of nuance I want to add to this picture, because they are important for understanding how this stack might evolve.
First, we have the analytic development process.
This is essentially the feedback loop that ensures that the credit decisioning stack continues to perform as well as possible for lenders.
The lender furnishes customer repayment data to the credit bureaus, which incorporate that data into the customer’s credit file. And the credit bureaus periodically license analytic providers (like FICO) a “development file” for them to use to refine and update their attributes and scoring models. These development files typically include millions of de-identified consumer credit files — every tradeline, inquiry, and public record, plus 24 months of forward performance.
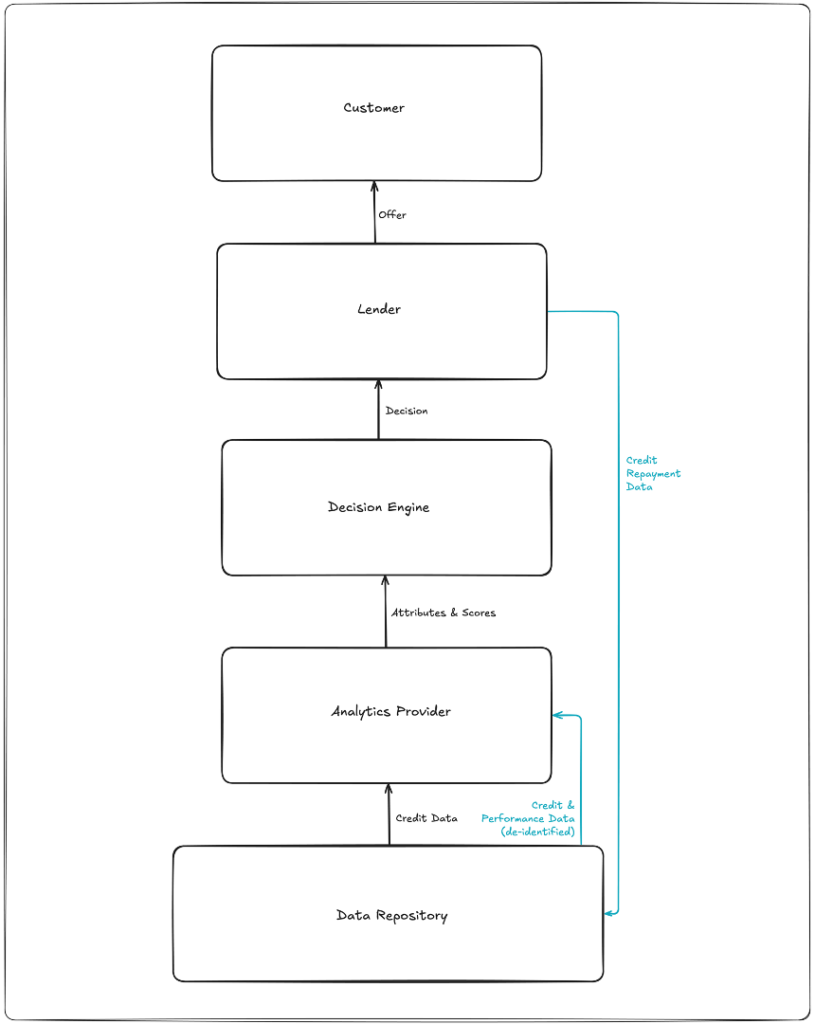
Second, we have customer visibility and control.
In the U.S., if a consumer is declined for a loan, they have the legal right to know why. This requirement manifests in the form of an adverse action notice (AAN), which is an explanation that a lender is required to give to a consumer if they deny the consumer for a credit product or take other “adverse actions,” such as offering them less favorable terms. In AANs, lenders are required to disclose their “principal reasons” for taking an adverse action.
The challenge, of course, is that it’s not always easy for a human being to be able to identify the “principal reasons” that an adverse action is taken in the credit decisioning process, especially when that process is governed by a statistical model (i.e., a credit score). To help with this challenge, the providers of those models began to provide lenders with “reason codes,” which pinpoint the specific factors that most contribute to a low credit score (and thus a decline).
The other big legal right that American consumers have is the right to see a copy of the credit data (typically in the form of report with summarized attributes) that was used to make a lending decision about them, to dispute any inaccuracies in that data, and to have their disputes investigated and (if they are proven correct) to have the inaccurate data corrected in a timely manner.
This dispute resolution requirement falls on the data repositories, which are companies that assemble and evaluate the consumer data that lenders use to make credit decisions. These companies are commonly called credit bureaus, but the technical term for them (as defined by the Fair Credit Reporting Act or FCRA) is Consumer Reporting Agencies (CRAs).
It’s important to note here that the boundaries between the different layers of the stack are not impermeable. Most CRAs provide at least some analytics (the big three credit bureaus have their own general-purpose credit score called the VantageScore), and some of them provide robust decision engines as well. This vertical integration of the credit decisioning stack can be very valuable for CRAs because it allows them to package their core data assets (which are largely commoditized) with complementary and more differentiated analytic and decisioning products.
Analytics providers will take a similar approach, though they will typically only bundle upwards (adding decisioning and loan origination software), rather than trying to compete with the data providers below them. This is because it’s much less expensive and annoying to act as a “technical service provider” working on behalf of lenders to analyze data and execute decisioning logic than it is to operate as a CRA (with all the attendant requirements).
This is the privileged position that FICO is in — no CRA obligations, but massive built-in distribution (the credit bureaus sell the FICO Score, alongside their data), access to ample de-identified performance data for attribute and model development, and the ability to expand into decisioning technology and other related lending software products.
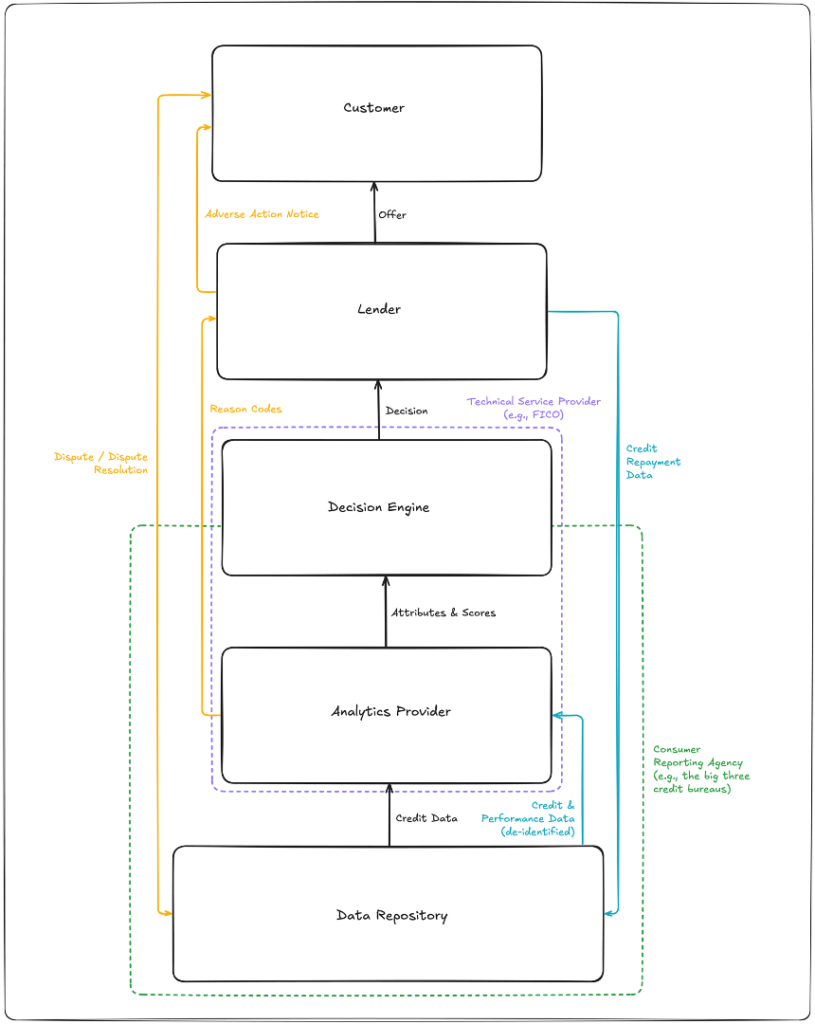
So, with that context in mind, how does the picture change when you add consumer-permissioned cash flow into the mix?
How Cash Flow Data Upends the Traditional Credit Decisioning Stack
The most important difference between cash flow-based credit decisioning and traditional credit decisioning, from an infrastructure perspective, is the origin of the data.
Rather than being furnished by lenders on a recurring cycle without consumers’ knowledge or permission, cash flow-based credit decisioning (in a modern open banking context) is entirely dependent on consumer permission.
Data aggregators, such as Plaid, MX, Finicity, and Akoya, collect consumers’ authorization and bank account credentials, pull their bank transaction data in real-time, and pass it along to the lender (or the lender’s technical service provider) for analysis and decisioning.
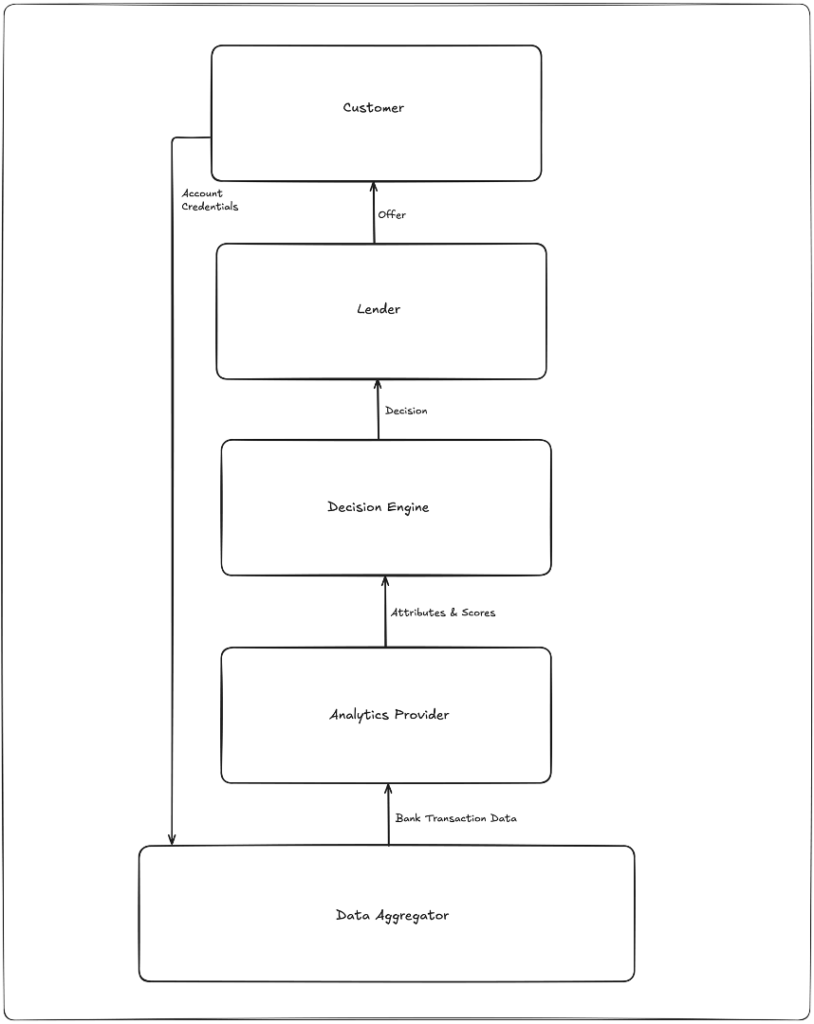
This is a significant change from the status quo!
Unlike the traditional credit bureaus, the data aggregators in this model are stateless. They don’t store consumer data. They simply pull the data, using the consumers’ credentials, clean and categorize it (raw bank transaction data is very messy and difficult to parse), and pass it along to the systems that will analyze it and decision off it.
This has a couple of important implications.
First, the consumer dispute and resolution process, which is governed by the FCRA (a law written 30 years before Yodlee, the pioneer in data aggregation, was founded), isn’t a super clean fit.
Traditional credit bureau data is riddled with errors. The bureaus have thousands of companies that furnish data to them every month, and, historically, the bureaus have taken a relatively hands-off approach to monitoring and regulating these furnishers. The result is that, according to a 2013 Federal Trade Commission study, one in five consumers have an error on at least one of their big three bureau credit reports, and one in twenty have an error significant enough that it could “lead to them paying more for products such as auto loans and insurance.”
This is why the right to review credit reports for free and to correct errors is so essential. There are a lot of errors, and, for the most part, they are quite obvious to consumers.
Cash flow data, by contrast, is much less likely to be incorrect in its raw form because it is sourced directly from the consumer’s bank account, and, to paraphrase one of my favorite Jerry Seinfeld jokes, if your bank transaction data is wrong, maybe getting declined for a loan isn’t your biggest problem right now.
The spot where errors can creep into cash flow lending isn’t in the raw data. It’s in the data cleaning and categorization process. For example, a data aggregator or analytics provider might incorrectly categorize a refund credit from Amazon as income or count a payment to McDonald & Sons Dairy Farm as a fast food transaction.
These types of errors are rarer, but (arguably) more difficult for consumers to detect when reviewing the cash flow equivalent of their free credit report.
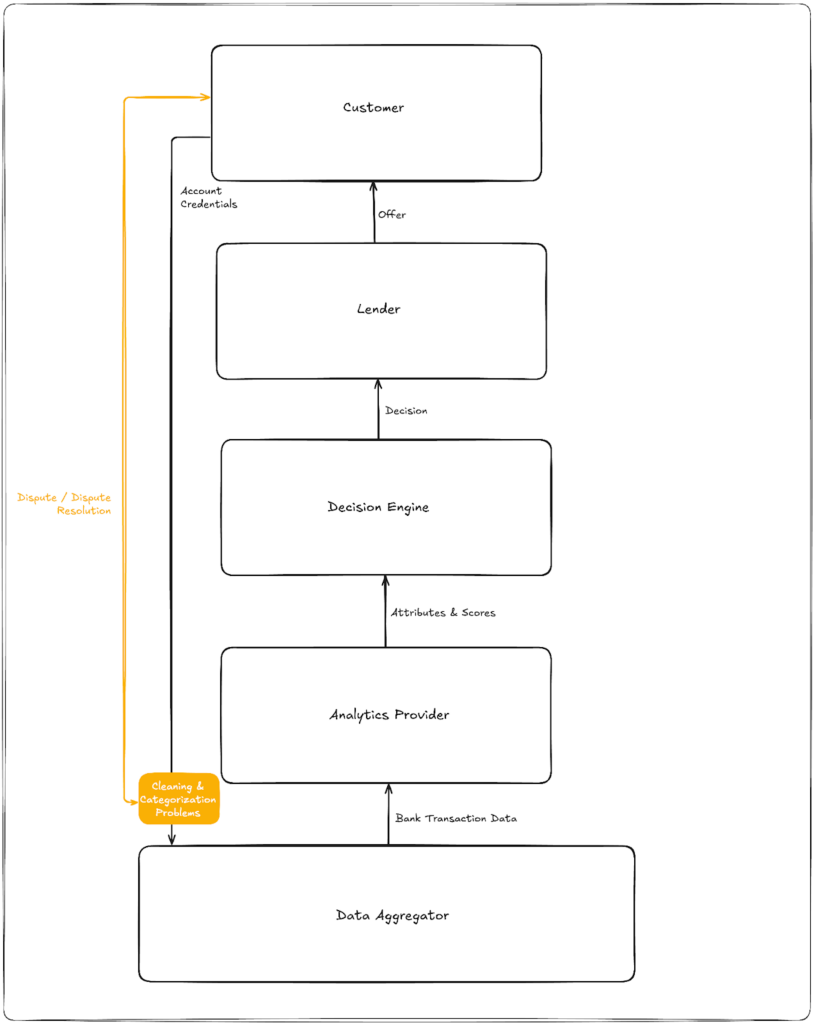
Second, the stateless nature of data aggregators makes it more challenging for those developing cash flow attributes and scores to access the data that they need.
Traditional credit data is a data scientist’s dream.
Every tradeline carries a monthly status code (current, 30–59 days past due, 60 days past due, charge-off, bankruptcy, etc.). Those same codes become the target variable for supervised learning, so a single file delivers both the predictors (balances, utilization, inquiries) and the performance label (“good” or “bad”).
Lenders furnish updates to the big three credit bureaus every 30 days in a standardized format (Metro 2), giving developers a clean time series that stretches back decades. And because virtually every mainstream creditor furnishes data to the bureaus, they can license de-identified development files containing tens of millions of consumers that mirror the entire market, reducing sampling bias and boosting out-of-time stability.
By contrast, cash flow data has some challenges.
Bank transaction data has no baked-in “paid/defaulted” signal. It doesn’t tell you how a loan performed. To be able to train a model, a data scientist needs to hunt down the corresponding loan performance data (from a lender’s servicing tape or a traditional credit file), marry it up to the snapshot of the customer’s cash flow data at the time of application, and then wait 12-24 months for the performance data to mature.
Additionally, the lack of a deep backbook of historical cash-flow data (like they have in traditional credit files) means that your model will have a limited view into seasonality (holiday spend spikes, tax refund inflows, etc.) and it will make out-of-time validation tricky because the training data won’t cover a complete economic cycle.
These challenges are addressable. Analytics providers can assemble consortiums of lenders (typically their clients) to provide the necessary performance data, but it’s difficult and time-consuming.
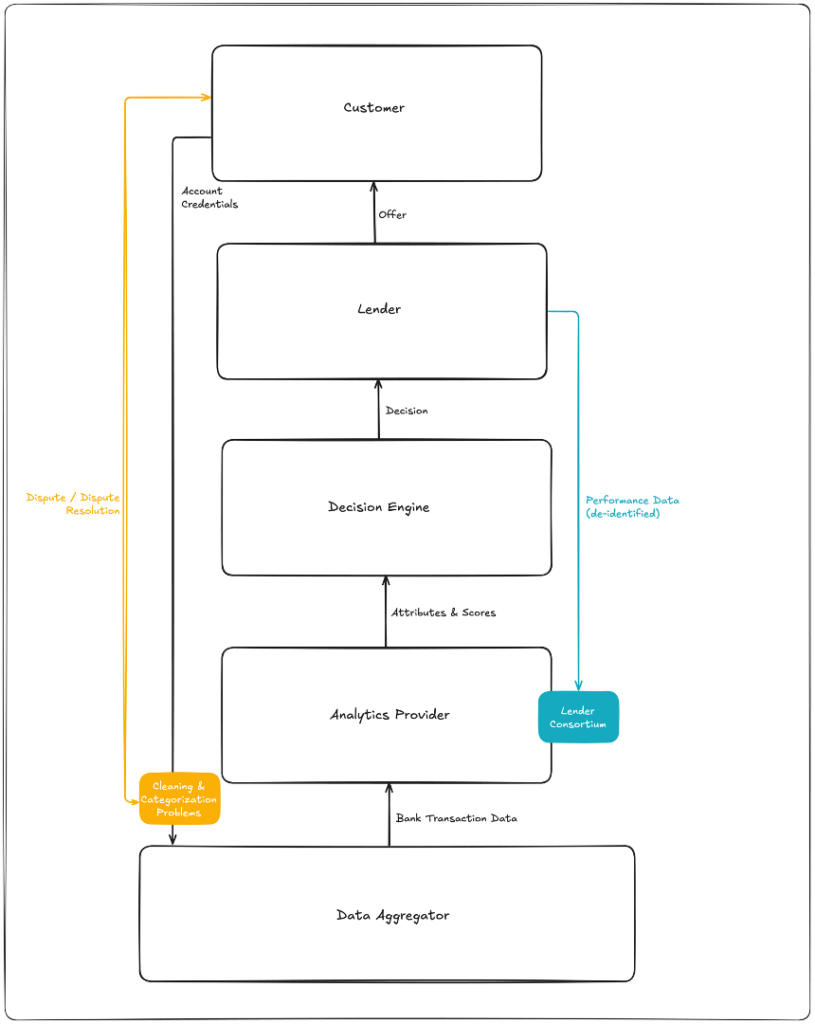
Given these differences between traditional credit data and consumer-permissioned cash flow data, it seems reasonable to assume that the “FICO of Cash Flow Data” (i.e., the best spot in the stack to capture the maximum amount of value with the least amount of cost and risk) might look a little different than FICO.
I don’t think anyone is in a position, right now, to say precisely what that opportunity will look like in the world of cash flow lending (or, indeed, if the same generational opportunity that was available to FICO in the 1990s will be available to anyone in the 2020s).
That said, there are a few companies out there that are actively trying to figure it out.
A Quick Survey of the Current Cash Flow Lending Analytics Ecosystem
Let’s wrap up by quickly talking about some of the leading providers in the cash flow lending analytics ecosystem.
Prism Data
Prism was spun out of Petal, a subprime credit card provider, in 2023. Petal’s long experience in cash flow underwriting, dating back to 2016, gave Prism a strong head start in developing its flagship CashScore.
Since then, Prism has assembled a consortium of third-party clients that share historical and ongoing performance data with them (they currently have roughly 10 million cash flow records). This widening base of cash flow and performance data underpins the company’s newer models.
Prism is probably the closest analog to FICO, in terms of its position in the stack. It’s not a CRA. It focuses exclusively on providing cash flow attributes and scores, and it (like FICO) has a growing number of distribution partnerships with traditional CRAs (Equifax, LexisNexis) and new CRAs (Plaid).
Nova Credit
Founded in 2016 to translate foreign credit files for U.S. immigrants, Nova broadened its focus to include cash flow analytics with the launch of its Cash Atlas product in 2022.
Like Prism, Nova uses cash flow and performance data received from its clients to develop and refine its attributes and models. Their consortium includes a wide range of lenders across different product categories, including credit cards, BNPL, auto loans, and personal loans.
Unlike Prism, Nova is focused on owning a bigger portion of the stack. In addition to its analytics, Nova has been a CRA for 7+ years, giving it a significantly longer track record operating as a “cash flow bureau” than most of its peers. It has also recently made a push to unify all its data acquisition, orchestration, and analytics capabilities within a single platform, creating a robust and compliant environment for lenders to operationalize a variety of non-traditional, FCRA-compliant data sources, such as cash flow, payroll, and international bureau data.
Edge
Edge has a similar origin story to Prism Data. It is an offshoot of a subprime lender called Credit Ninja, with early versions of its attributes and scores built primarily on Credit Ninja’s performance data.
Today, Edge, like Nova Credit, is both an analytics provider and a CRA (which it became in 2023). However, unlike Nova (or Plaid or Finicity), Edge is not a stateless cash flow bureau. In addition to pulling in consumer-permissioned bank transaction data using the consumer’s bank account credentials in real-time, Edge also maintains a data lake of historical cash flow data (stored from previous transactions where consumers provided their credentials) and credit repayment data provided by its clients.
This unique approach gives Edge a significant depth of data for attribute and model development (though still confined to the lending products and customer segments targeted by its clients), as well as the ability to offer data products to its clients that do not require explicit consumer consent or credentialing (such as lead screening). It’s unclear to me how compatible this approach is with the rigid constraints of the CFPB’s Personal Financial Data Rights Rule, as currently written, especially the restrictions on secondary data use.
Experian
We all know Experian, obviously.
However, it bears mentioning here because it is the only one of the big three credit bureaus to evince a significant interest in competing in the growing cash flow lending analytics market.
This isn’t surprising to me. Experian has always been the most innovative and analytically inclined of the big three. What’s interesting to me is how the company is choosing to pursue its cash flow ambitions.
Its newest product in this area is the Cashflow Score, a cash flow-based credit score that ranges from 300 to 850.
As a traditional credit bureau, Experian has an enormous built-in advantage in building cash flow attributes and scoring models using its wealth of historical credit performance data. Curiously, however, Experian is not choosing to double down on that advantage in how the Cashflow Score is being implemented. From Experian’s press release (emphasis mine):
Experian’s new Cashflow Score leverages consumer-permissioned transaction data provided by its clients. From there, Experian, acting as a technical service provider on behalf of its clients, categorizes the transaction data and calculates attributes that are used to derive the score, which is delivered back to the lender.
A technical service provider! Not a CRA!
As I wrote about last month when this product was first announced, that’s weird! It makes sense for a company that isn’t a CRA (like Prism Data or FICO) to avoid becoming a CRA if it doesn’t feel the need to. Being a CRA is expensive and frequently unfun (unless you enjoy constant scrutiny from regulators and consumer advocacy groups).
But Experian is already a CRA! They already pay the price for being one of the biggest CRAs in the country! Why not implement your Cashflow Score in a way that leverages your status as a CRA to create competitive differentiation? Why, for example, wouldn’t you create the ultimate blended credit score, combining traditional credit data and cash flow data, in much the same way that they combine traditional and alternative credit data in their Lift Plus and Lift Premium scores? You already have Experian Boost, which leverages consumer-permissioned bank account access to identify and modify consumers’ core Experian credit files!
The only thing I can figure is that Experian is nervous about the idea of fulfilling the duties of a CRA (particularly around disputes) when cash flow data is used to decision (and decline) consumers for credit. So, instead, it’s trying to be the FICO of cash flow lending.
Fascinating.
Where Could This Go Next?
One of the subtle, but extremely important advantages that the FICO Score had, as it was cementing its dominant position in the market, was access to data.
By 1989, the big three credit bureaus had assembled the perfect laboratory for the development of a general-purpose credit score. A nationally representative and historically rich sample of repayment snapshots and loan outcomes in a single schema, refreshed monthly, and already reconciled to regulatory disclosure requirements.
FICO stepped into that lab, built a great score, and somehow convinced the lab owners to sell it to other data scientists while also allowing FICO to continue using the lab to improve their product!
By the time the big three credit bureaus woke up and realized the strategic mistake they had made (they launched VantageScore in 2006), it was too late. FICO was too entrenched. There was no way to kick them out of the lab!
Cash flow analytics providers are not nearly so well-positioned.
As we’ve already discussed, cash flow data is inherently more challenging to develop models off of because it doesn’t have built-in performance data. You have to go out and get it. And my educated guess is that the fight for that data will become significantly more intense over the next 5-10 years. Lenders participating in different data-sharing consortiums will have a lot of leverage, as providers fight to expand the scope and coverage of their proprietary training data.
Plus, unlike the blissful ignorance of the late 1980s and 1990s, everyone in the ecosystem today knows how valuable it will be to have the best cash flow attributes and scores. We will see more competition. And some of it may be quite creative.
I was talking to my fintech friend Tim Bates (author of an incredible research report on cash flow underwriting BTW) about this, and he suggested a few intriguing competitive possibilities:
- An “On Us” Data Consortium. Build a consortium of big banks that provide historical snapshots of their “on-us” deposit data. This would have the advantage of not being stateless, and would be reasonably accurate as a historical record or proxy for model building. The big banks could also furnish de-identified performance data to marry with the transaction data. This would likely require a bank-owned consortium to pull it together (big banks aren’t naturally good at working together), but luckily, a jointly owned bank venture in the open banking space already exists!
- A FICO Credit Union. What if, as a consumer, you could monetize your own data and pool it with other like-minded individuals who want to benefit each other cooperatively? It’s not that different from starting a credit union, but instead of pooling the group’s money to lend it out, they’re pooling their data to create an advantage that each member as an individual couldn’t scale up to on their own. Consumers’ data is an asset, but unlike cash, they can give it or sell it to another party and still retain ownership over it. Think of it as the credit union equivalent of FICO. You join the cooperative and can opt your data in for various projects that you see as either aligning with your values or your commercial interests. If you share your data as part of the cooperative effort and it pays off as a revenue-producing asset (e.g., a model) that can be sold to lenders, then you benefit from it financially as a member of the cooperative.
Some of this might sound a bit crazy, but trust me — the disassembly and reassembly of the U.S.’s credit decisioning infrastructure is going to inspire some crazy ideas.